Speaker: Emanuele Rossi (Imperial/Twitter)
Problem: Many Graphs are Dynamic
E.g. social/interaction networks
- How do we make use of timing information to make better representations of nodes?
- Can we predict when and how the graph will change in the future?
This talk focuses on the first question.
From static to dynamic graphs
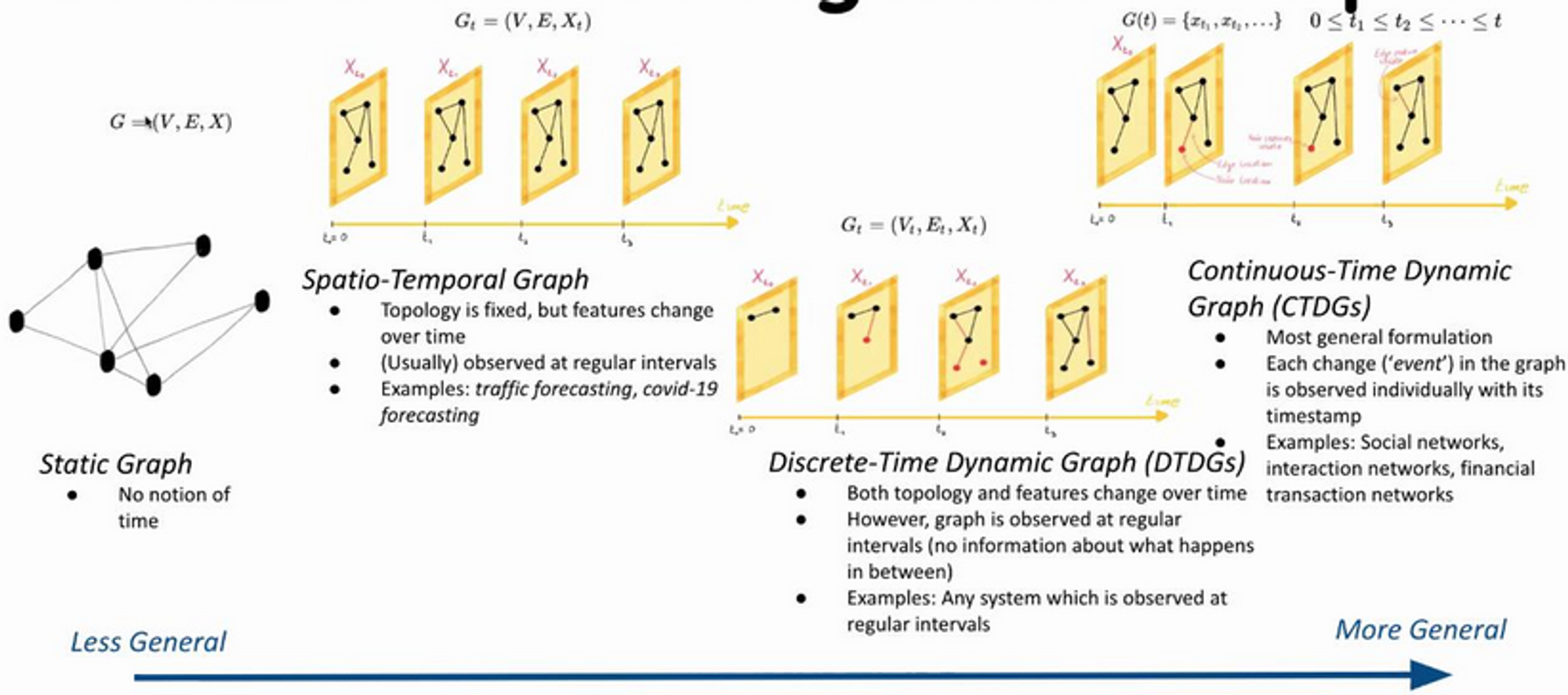
CTDGs: many types of events
Node/edge - creation/deletion/feature change
Why is learning on dynamic graphs different?
Model must:
- Handle different kinds of events
- Use time information
- Efficiently incorporate the graph changing - don't recompute everything
- Different tasks: predict when something will happen
Model

Encoding a temporal graph
Simplification: assume the graph consists entirely/only of edge creation events
First idea: Process events in order using an RNN with a different hidden state for each node
Pros: Built in bias of sequentiality
Cons:
- Not using the graph of interactions directly
- Memory staleness: nodes only updated when involved in interaction
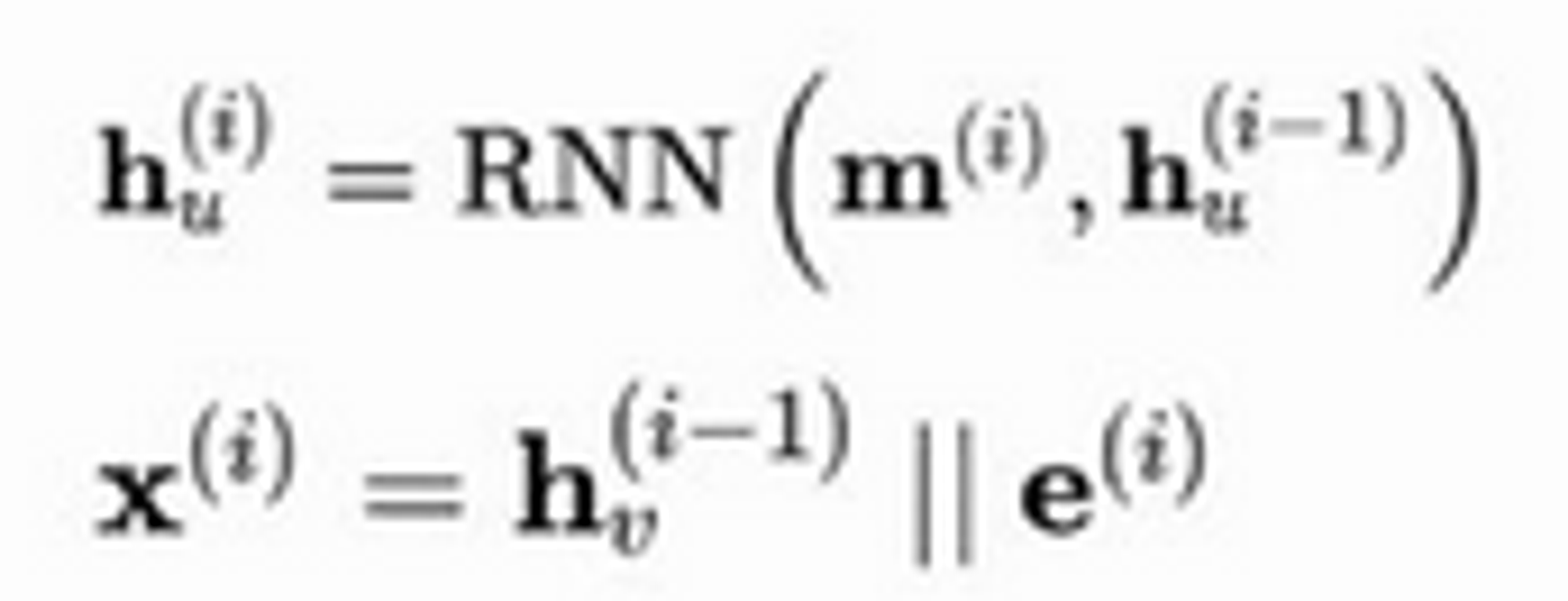
RNN for a node takes previous state, plus message
Second idea: Use a GNN with attention and use timestamps as edge features
Pros:
- More efficient - no need for sequential processing
- Uses the graph explicitly, mitigates staleness because neighbours will still be updated
Cons
- Can only handle edge addition events
- Not suitable for online updates

Temporal Graph Networks
Attempts to combine best of both worlds
- Defines general framework which can handle all types of events
- Each type of event generates a message used to update node representations
- Uses GNN directly on graph of interactions
Module 1: Memory
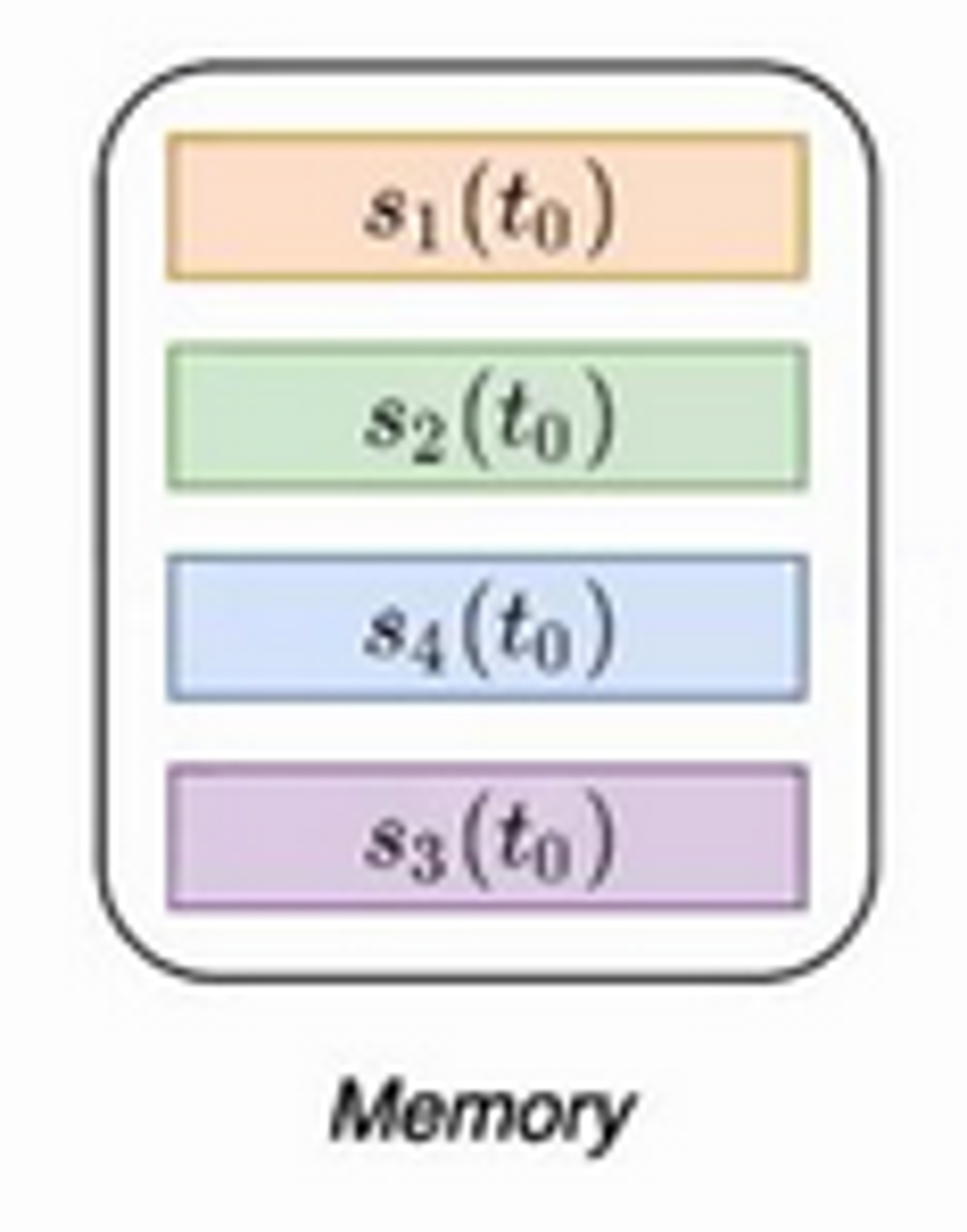
- One per node
- Analogous to RNN hidden state
- Contains a vector for each node the model has seen so far—a compressed representation of all past interactions of a node
- This is not a parameter - it is also updated at test time
Module 2: Message Function
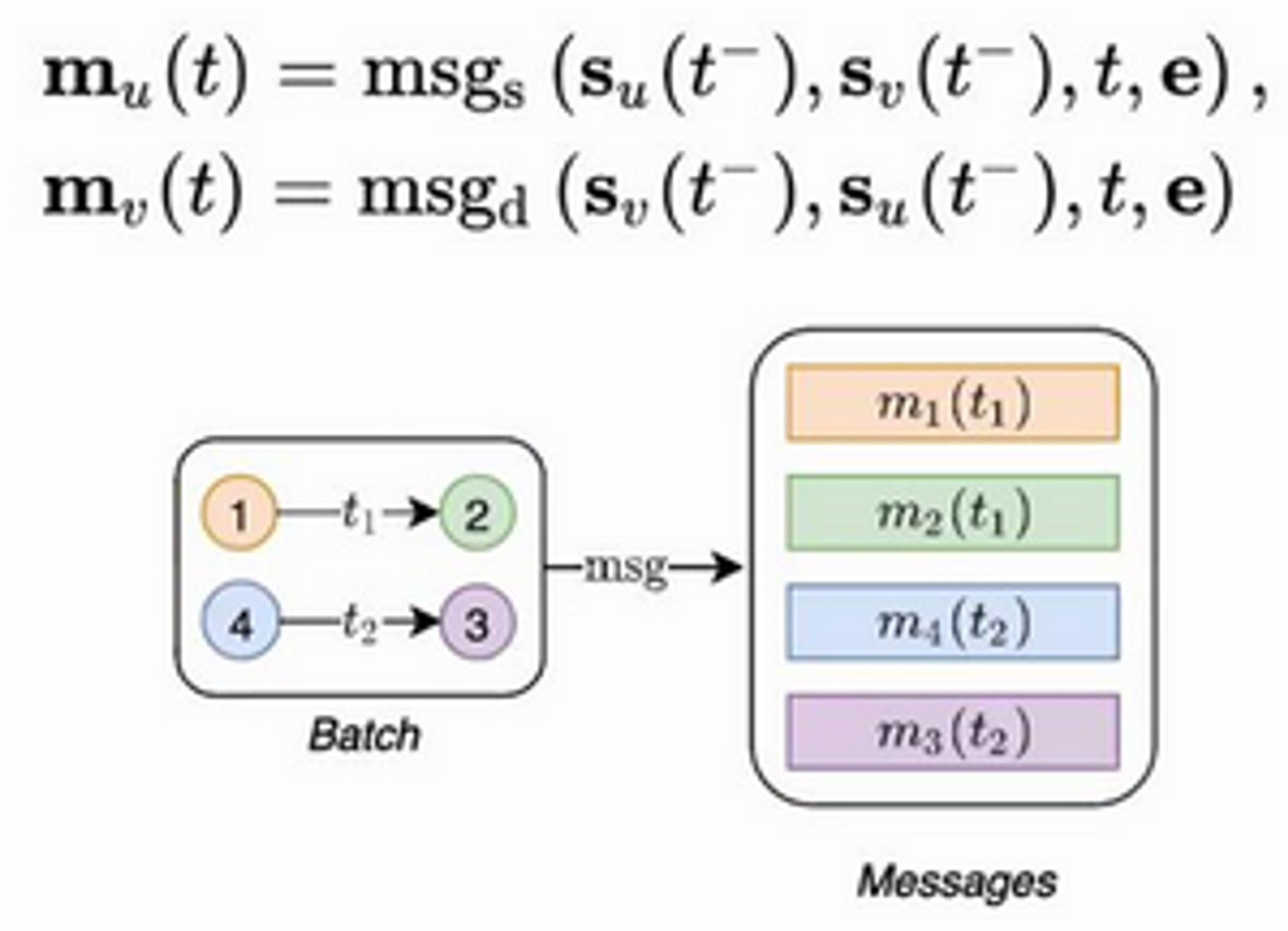
- Each event creates a message
- Messages used to update memory
- Given an interaction , messages are computed
- This is done for source and destination
Module 3: Memory Updater
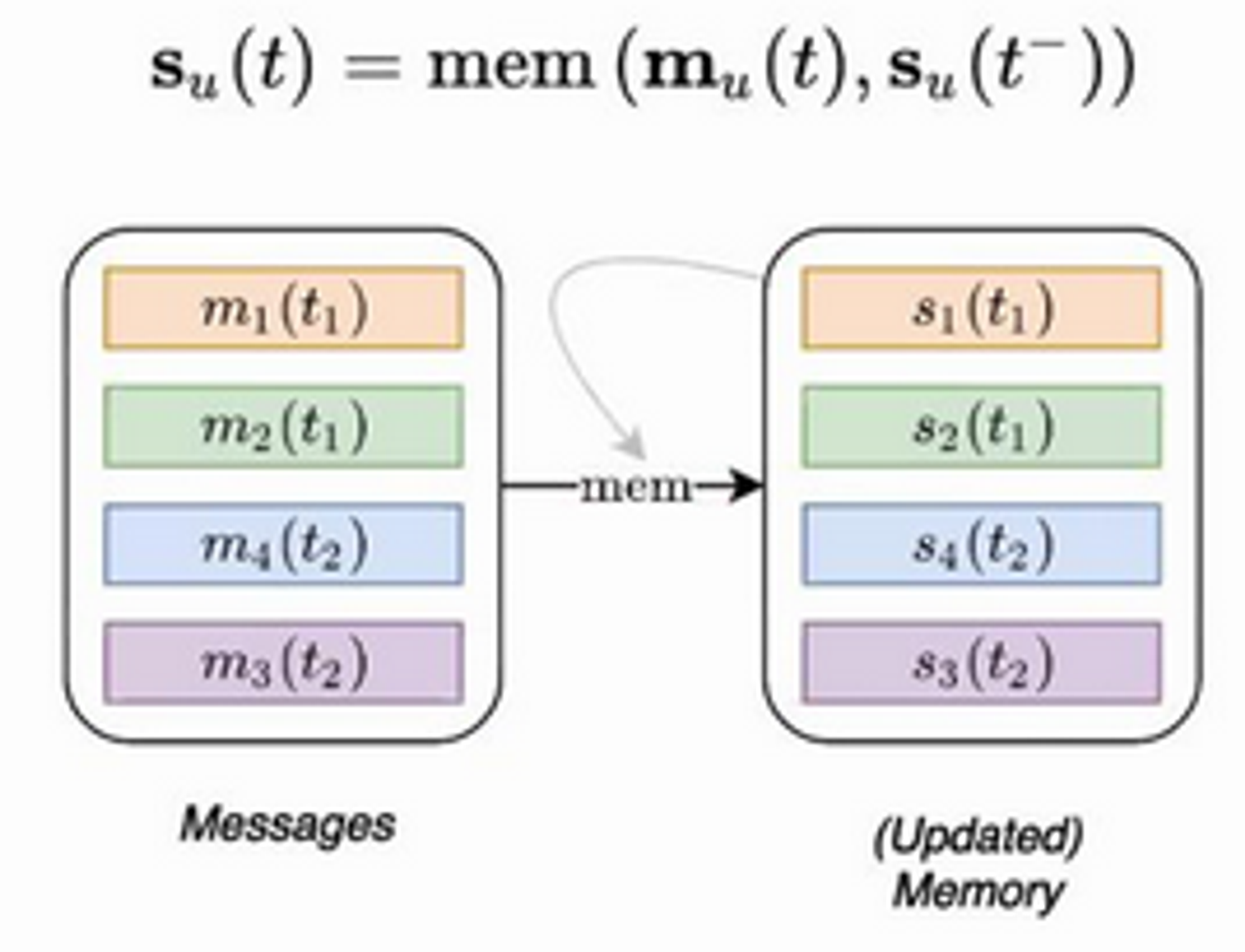
- Memory updated using newly computed messages
- This is an RNN (GRU used)
Module 4: Graph Embedding
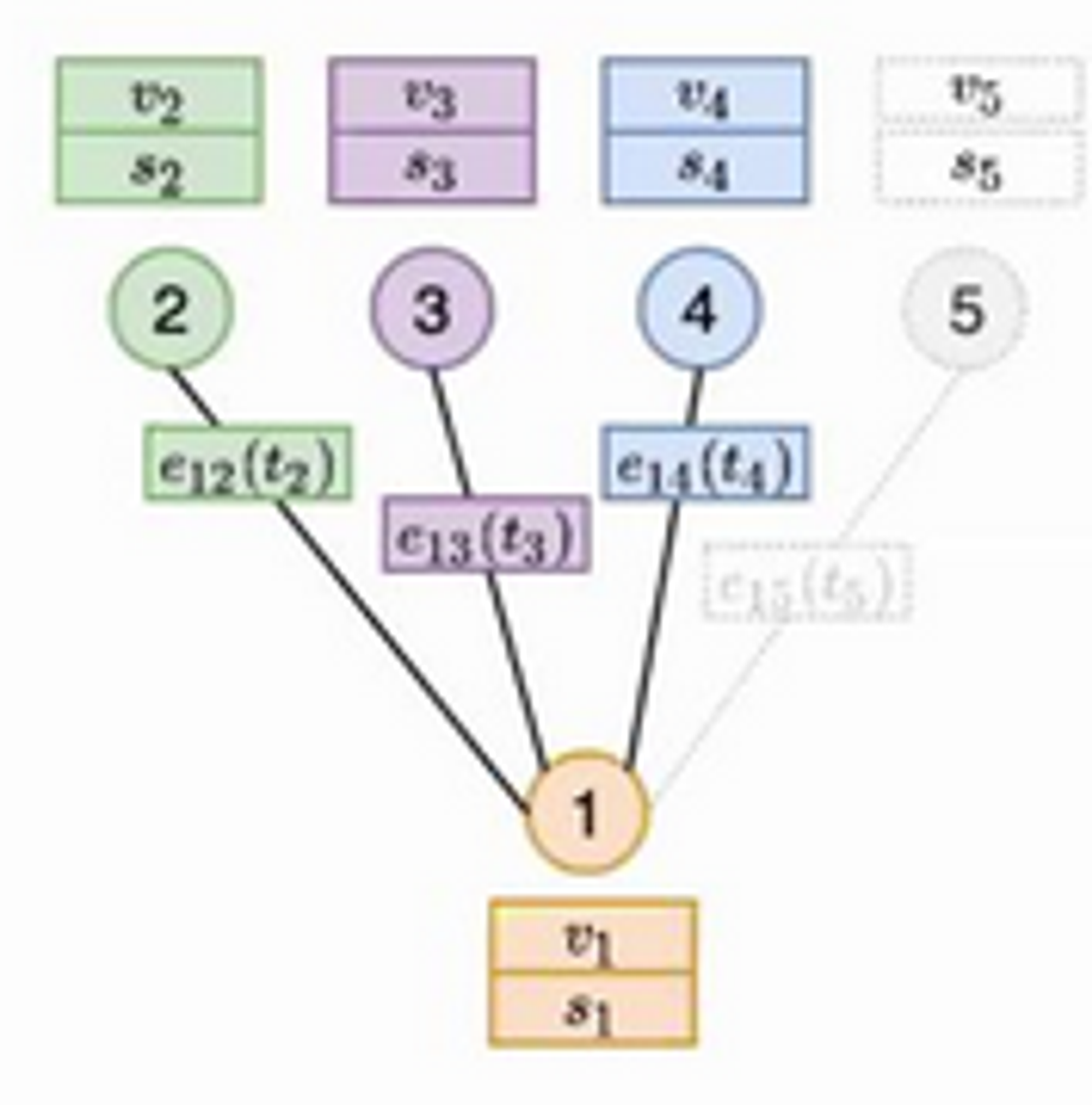

- Adding a GNN over everything from previous modules
- Essentially, a GNN is run over the current graph, using the memory as additional features
- Uses graph attention over node features and memory
Link Prediction with TGN
- Data split is chronologically
- All previous events used in prediction of next one
- Training this efficiently is not trivial - see paper for details!
Scalability
- Memory is a concern for scalability as it is not a parameter → we can just think of it as an additional feature vector for each node
- So we only ever need memory for nodes involved in a batch
- Model is scalable as GraphSage → can scale to very large graphs!
Experiments
Link prediction:
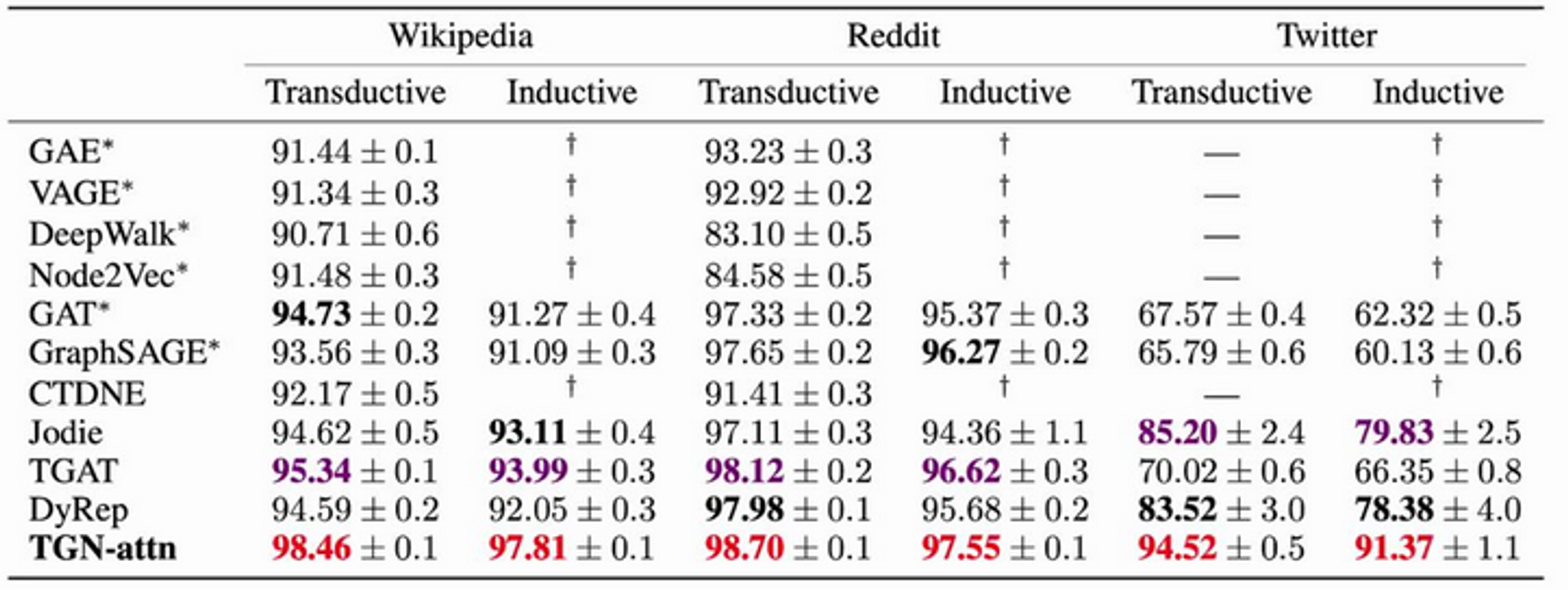
It's not clear to me how they've wired up the non-temporal methods here!
Other experiments also presented. Performance similarly strong.
Ablation study also included. Memory and embeddings both very important.
Q & A
- Timestamp representation is a difficult problem - some work has been done on this. Raw timestamps are bad, embeddings a bit better. More work needed though!